Ask Data Center Frontier: Parsing the Logistics Equation from Hyperscale to the Edge
Question: Is logistics any more integral to the outgrowth of edge/distributed computing for AI than it is for other data center segments, such as hyperscale or colocation? Is it a case of apples and apples, or apples and oranges?
Answer: The rise of edge computing for AI introduces distinct logistical challenges compared to hyperscale data centers. While hyperscale facilities prioritize centralized, high-capacity deployments with predictable supply chains, edge AI requires a distributed footprint—often in urban or remote locations with constrained space and power.
This demands agile logistics for deploying modular infrastructure, managing last-mile connectivity, and maintaining equipment across hundreds or thousands of sites. The need for low-latency processing also pushes edge deployments closer to end-users, adding complexity in site acquisition, permitting, and energy sourcing—factors less pronounced in sprawling hyperscale campuses.
Yet the core principles of scalability and efficiency still apply. Both segments rely on robust supply chains for hardware, but edge AI’s smaller-scale, decentralized model amplifies the stakes for logistics precision. Hyperscale operators can absorb delays with buffer inventory; edge networks, however, face compounding risks if deployments lag or fail.
The result? Edge logistics isn’t just about moving gear—it’s about synchronizing a patchwork of localized solutions while maintaining hyperscale-like reliability. In that sense, it’s less "apples versus oranges" and more a question of grafting hyperscale rigor onto a far more fragmented orchard.
Logistics in Hyperscale vs. Edge AI Infrastructure
Hyperscale Data Centers:
Logistics for hyperscale data centers centers around managing the vast infrastructure required for massive deployments of servers. This includes coordinating power, land acquisition, fiber infrastructure, cooling systems, and supply chains to support thousands of servers housed in centralized locations. The primary challenge in this segment is speed to market for large-scale projects. Providers must navigate complex land constraints, secure regulatory approvals, and ensure high-capacity power provisioning to meet growing demands.
Once a hyperscale facility is operational, the focus of logistics shifts from the physical movement of components to managing long-term supply chain stability. This includes ensuring consistent component availability, sourcing sustainable materials, and maintaining a stockpile of spare parts to support ongoing operations.
Edge/Distributed AI Infrastructure:
In contrast, edge and distributed AI infrastructure require a decentralized logistics model. Rather than consolidating operations into large-scale data centers, these networks often deploy smaller compute units across many different sites. The challenge in this model lies in scalability at the edge, where real estate, power, and connectivity can vary greatly from site to site. Managing the complexities of infrastructure deployment at numerous locations requires agile logistics operations and careful planning.
Adding to the complexity is the need for frequent equipment refresh cycles to keep pace with evolving technologies. These rapid refresh requirements, coupled with the need for fast deployment across diverse and often unpredictable environments, create additional logistical hurdles that require careful coordination and flexibility.
Where Logistics Becomes More Integral to Edge AI
As edge AI infrastructure continues to proliferate, logistics becomes a critical component for managing diverse and often unpredictable environments. Unlike hyperscale data centers, which can benefit from centralized, bulk operations, edge AI deployments require more nuanced and flexible logistical strategies. Here’s where logistics becomes more integral to the success of edge AI infrastructure:
• Diverse Locations: Edge AI deployments require navigating everything from urban rooftops to retail backrooms, telco sites, and industrial hubs—each with distinct permitting, power, and cooling constraints.
• Hardware & Maintenance Complexity: Unlike hyperscale, where logistics supports bulk shipments and on-site spares, edge deployments require just-in-time hardware delivery, remote management, and often on-demand servicing.
• Supply Chain & Network Coordination: AI workloads demand high-performance GPUs/accelerators, which are already supply-chain constrained. Distributing these components efficiently across many sites is a unique logistical challenge compared to centralizing them in a hyperscale environment.
• On-Site Energy Considerations: Edge sites may require on-site power solutions (e.g., microgrids, batteries, fuel cells), which adds another logistical layer in sourcing and maintaining diverse energy solutions across many locations.
Adding Colocation to the Data Center Logistics Equation
To complete the picture, it’s also important to consider where colocation fits into the logistics equation—offering a middle ground between the centralized scale of hyperscale and the decentralized complexity of edge AI.
Colocation facilities sit somewhere between hyperscale and edge in terms of logistics complexity. They are typically regional or urban, located in established data center markets with mature infrastructure and well-defined delivery routes.
Equipment deployment is largely predictable, with logistics focused on racking, cabling, and integration within existing multi-tenant environments. Ongoing logistics are supported by staffed facilities, structured SLAs, and ready access for client technicians or service partners.
Where Colocation Differs Logistically from Edge AI:
-
Centralization & Predictability: Colocation sites consolidate many customers under one roof. This reduces logistical fragmentation compared to edge AI, which may deploy single-purpose infrastructure across dispersed locations.
-
Standardized Environments: Logistics at colocation sites benefit from consistency—same rack types, same access controls, same power distribution—which simplifies delivery, installation, and service.
-
Staffed Support: Most colocation facilities have remote hands or on-site technicians available, eliminating the need for traveling field teams to handle troubleshooting, upgrades, or maintenance.
-
Lower Deployment Urgency: While edge AI often competes on speed-to-deploy for latency-sensitive apps, colocated workloads are more flexible in terms of proximity to users, so deployment logistics are more routine.
Conclusion
It’s not apples to oranges, but it’s definitely Gala vs. Granny Smith vs. Fuji—involving the same fundamental supply chain concerns; but edge AI’s distributed nature makes logistics a far more dynamic and site-specific challenge compared to the bulk efficiencies of hyperscale or the standardized, centralized model of colocation.
As AI infrastructure expands into all three realms, logistics isn’t just a backend concern—it’s emerging as a core enabler of deployment strategy, especially where speed, scale, and locality intersect.
At Data Center Frontier, we’re committed to exploring where the data center industry is headed—and helping our readers navigate that journey with clarity and confidence. Our new feature, "Ask Data Center Frontier," invites you to be part of the conversation.
Backed by editorial insight and industry expertise, this column is designed to shed light on the trends shaping the future of digital infrastructure. Each installment will address timely, thoughtful questions in the sector, on topics ranging from site selection and power strategy to AI infrastructure and operational best practices.
Have a question you’d like us to explore? Type "Ask DCF" in the Subject line and send it to Editor in Chief Matt Vincent: [email protected].
At Data Center Frontier, we talk the industry talk and walk the industry walk. In that spirit, DCF Staff members may occasionally use AI tools to assist with content. Parts of this article were created with help from OpenAI's GPT4.
Keep pace with the fast-moving world of data centers and cloud computing by connecting with Data Center Frontier on LinkedIn, following us on X/Twitter and Facebook, as well as on BlueSky, and signing up for our weekly newsletters using the form below.
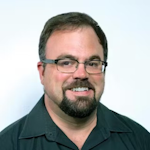
Matt Vincent
A B2B technology journalist and editor with more than two decades of experience, Matt Vincent is Editor in Chief of Data Center Frontier.