Natural Language Processing: The Bridge Between Man and Machine
Artificial intelligence (AI) is really an umbrella term which encompasses a range of other technologies. One of those components is natural language processing (NLP), which can create intelligent interactions between machines and humans. To fill the gap between people and machines, NLP leverages code, computational linguistics, and even computer science to help understand and even manipulate human language.
This type of technology allows for the comprehension by computers of the structure, meaning, and composition of various human languages. From there, it allows users (and machines) to interact with other computers and systems using natural sentences. Examples of NLP in action include intelligent assistants like Apple’s Siri, Microsoft’s Cortana, and the Amazon Echo (Alexa). Tyhese devices are leading to a dramatic increase in the use of voice as an interface, a trend that appears likely to continue as marquess tech firms use NLP to voice-enable new products.
It’s no wonder that we’re seeing a vast boom in NLP solutions. These types of cognitive systems can greatly step up the frequency, flexibility, and immediacy of data analysis across a range of industries, circumstances, and applications. In a recent report, IDC estimates that the amount of the global datasphere subject to data analysis will grow by a factor of 50 to 5.2 zettabytes in 2025; the amount of analyzed data that is “touched” by cognitive systems will grow by a factor of 100 to 1.4 ZB in 2025.
Natural language processing allows us to understand meaning behind various speech processes. The resulting capabilities can include sentiment analysis, or the “voice” of the customer, speech inference, relations and patterns between people and entire social groups, and even variations in market trends.
NLP Systems: Impacting Data Center and the Cloud
As new technologies emerge in our industry, don’t think that they’re confined to cloud systems. You’ll see a lot of new offerings coming from the big three cloud vendors, but it’s important to note that colocation and data center providers are also working with new solutions which can support advanced cognitive technologies.
That said, there are a few areas to focus on when it comes to working with solutions like NLP, cognitive systems, and data in general.
- The vast majority of cognitive solutions, NLP included, will be low-latency applications. The entire goal is to process critical data points as close to the source as possible, and as quickly as possible. To an end user, latency is the reason that downloading a movie “takes so long.” But to a data center provider the number of milliseconds it takes to complete a function can be measured in customer dissatisfaction and even cost. For a business focusing on delivering cognitive solutions, latency can also mean the loss of a competitive edge. In working with things like NLP and data processing, high latency means that the sender spends more time idle (not sending any new packets), which reduces how fast throughput grows. Distance from the data source could also lead to packet loss. Even with 2% packet loss, TCP throughput is between 6 and 25 times lower than with no packet loss. When working with tasks like data ingestion and NLP solutions, be sure to fully understand network connectivity, where your data center might need improvements, and how to support advanced customer use-cases.
- I/O and infrastructure for cognitive solutions must be a serious consideration. Please note that services, functions, and even virtual machines running cognitive systems or NLP platforms are not your typical workloads. When working with data analytics, the expectation is to actually do something with the data in a timely and efficient manner. If you’re running a data analytics job on Hadoop, for example, this may be CPU, memory, or even I/O intensive. Similarly, if you’re running a machine learning engine for the purposes of NLP, you could very well require quite a few CPU cycles. Working with NLP real-time analytic could be super memory intensive, and if you need to transform or prep data for analytics you might have very I/O intensive workloads. The secret for the data center leader is in sizing and knowing your market. Are you going after a broad market or maybe just healthcare or retail, for example? Maybe you’re aiming your services at content and content providers. In designing your data center to support cognitive systems, conduct both a business and technology study to size your systems properly.
- Edge architectures are specifically designed to support data analytics and cognitive systems. Companies are trying to gain more “intimacy” with their customers through new types of services, applications, and the IoT (Internet of Things). IDC predicts that by 2020, the spend on edge infrastructure will reach up to 18 percent of the total IoT infrastructure spend. The “closeness” of these solutions will drive them to better deliver value when it is needed (right time, right place, right product). To generate this intimacy, proximity will be critical in order to manage the volume of information and generate a timely result. Enabling technologies like 5G wireless and edge computing are making this movement accelerate. And, this is also a major part of an organization’s digital journey. That is, to impact customers while still delivering powerful business value. Remember, the goal of edge computing is to allow an organization to process data and services as close to the end-user as possible. Depending on your use case and the market you’re targeting, working with edge might make a lot of sense.
- Creating the business differentiator between traditional and next-generation capabilities. This is going to be a very direct message to all of my friends in the data center, colocation, and provider space. Please, don’t make your cognitive solutions “paperware” consisting of marketing collateral and some PDFs and suggest that you have a cognitive-capable data center. The reality is that you’ll get customers, but they’ll lose faith quickly. This will impact your brand. If this is a road you’re going to go down, you need to have a deep understanding of the market and your own capabilities. This is a true moment for you to combine technology and business to go after a quickly emerging, data-driven market. Don’t be afraid to go after a niche. NLP capabilities for, let’s say healthcare, can really help with patient sentiment to help healthcare operators understand what their customers really want. Plus, many healthcare providers simply aren’t willing or ready to go to the cloud. This is where you come in!
Looking ahead
If you’re a data center operator or provider, and you’re looking to get into this space, my biggest piece of advice is to make sure you don’t take a traditional approach. These aren’t your typical workloads or VMs. They may require additional levels of redundancy, and you might need to deploy more cooling to dense systems, and you might need to look into better data distribution capabilities. Systems like edge can help you get closer to both users and data, but this can be an investment.
Remember, your customers are actively looking into NLP to help them create competitive advantages in a market that’s constantly changing. They can either go to the cloud, or one of your offerings. Make sure it makes sense, design around solutions that set you apart, and focus on best practices. Use cases around cognitive systems will only continue to grow as we continue to immerse ourselves in a data-driven world.
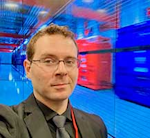