Cerebras and G42 Introduce First Piece of a Distributed AI Supercomputing Cloud
Working with their partner G42, a UAE-based technology holding company, Cerebras has launched the first node of their eventual 36 Exaflop AI training cloud cluster with the initial deployment of the Condor Galaxy 1 in the Colovore data center in Santa Clara, California.
Based around the Cerebras Wafer-scale Engine, technologies have been developed to allow the WSE to operate at cluster scale. First introduced in late 2022 in the Cerebras Andromeda AI supercomputer, this original implementation was deployment of a 1 Exaflop AI supercomputer using 16 Cerebras CS-2 systems.
More than simply a proof of concept, the Andromeda supercomputer was used to launch the Cerebras Cloud and enabled them to train seven Cerebras-GPT models in only a few weeks.
These models were then open sourced for worldwide use. This also allowed Cerebras to offer the services to their cloud customers, making the resource available to customers who no longer needed to invest in their own hardware.
Scaling Toward 36 Exaflops of AI Processing Power
The new generation Condor Galaxy 1 AI supercomputer expands on the impressive results seen in the scaling of the Andromeda system. With a plan to expand to nine nodes by the end of 2024, the goal is to offer a remarkable 36 Exaflops of AI processing power running at FP16 with scarcity.
The initial release of the Condor system, which is available for customer use via the Cerebras cloud, gives customers access to 2 Exaflop capabilities. This Phase 1 release is basically half of a fully completed Cerebras supercomputer instance.
A full instance is comprised of:
- 54 million AI optimized compute cores
- 82 terabytes of memory
- 64 Cerebras CS-2 systems
- 386 terabits of internal cluster fabric bandwidth
- 72,704 AMD EPYC Gen 3 processor cores
So, this initial release contains exactly half of that hardware. Phase 2 plans for a second set of 32 Cerebras CS-2 systems to be deployed by the end of this year will create the full 64 CS-2 AI supercomputer, running at 4 Exaflops.
These next 32 systems will be installed in the same data center as the existing install. The fact that the CS-2 nodes can be installed in a standard rack and draw only 30 kW of power simplifies the installation process.
Facing the GPU Bottleneck
Cerebras tells us that the timeframe from the start of the installation to training the first models is just 10 days. Andrew Feldman, CEO of Cerebras, pointed out that their biggest bottleneck in the introduction of additional systems is getting the WSE GPU, which is produced for them by TSMC, much like many other fabless designs.
The difference, of course, is that a single WSE, produced using a 7nm process, is a roughly 8.5” square piece of silicon, containing 850,000 processing cores optimized for AI and roughly 2.6 trillion transistors.
If you are wondering about the performance claim, Feldman tells us that the performance of the CS-2 instances is completely linear; each 64-node instance added to their cloud will bring an additional 4 Exaflops of performance. So the expectation is that Phase three, which will be a deployment in two additional data centers in the US, will triple the performance of the system, to 12 Exaflops by mid-2024.
While Cerebras has not announced the location of these next deployment sites, there have been reports that Asheville, NC is being considered for a new data center to house at least one supercomputer instance.
The final phase of the project, scheduled for completion by the end of 2024, will bring six additional instances online, presumably in a worldwide deployment to simplify global availability of the Condor Galaxy in the Cerebras cloud.
With this deployment, the Condor Galaxy, claimed by Cerebras to be the first fully distributed supercomputer network, will deliver its 32 Exaflops of performance, giving it a significant performance advantage over any other AI computing vendor’s announced supercomputing plans or existing infrastructure.
And keep in mind that this performance will be available as a public cloud computing resource.
Simplicity Is Key
Feldman also makes a point of the simplicity of building large models on their system, telling us that because of the way that the system scales, the same code that was written to run a 40 billion parameter model can effectively run a much larger model “whether you go to 100 billion or a trillion parameters, whether you're 40 or 60, or 100 machines, you don't have to write a line of extra code.”
The implication here being that if you want to increase the speed at which LLM training is being done, you can simply throw additional resources at it, without changing the code you’ve written to use that model.
Feldman points to the GPT-4 paper, where one citation mentions that 100 people were involved in the coding for a distributed compute model, and points out that for the Condor distributed supercomputer, it’s a half-day project for one person.
This works because the Cerebras Wafer Scale Cluster operates effectively as a single, logical accelerator. Memory is a unified 82 terabyte block, meaning no special coding or partitioning required; the largest model currently in use can be placed directly in memory. The same code works for 1 billion parameters and 100 billion.
If there is a downside to the Cerbras Cloud, it’s that it’s designed for big workloads. It simply isn’t efficient to use it for small models and small problems, for example, in the under 500 million parameter range.
Feldman uses an automotive metaphor: “A pickup is a lot of vehicle to go to the grocery store. You can fit that in a hybrid car. Don’t buy the pickup for grocery runs. If you're moving 50-pound bags of concrete and lumber then you buy a pickup. And that's how we fit in for problems that require more than eight GPUs. Below that, we're not a cost effective solution. But those 8 GPU nodes, that do a great job at 200 million parameters, get less cost-effective as you begin to cluster them. In the case of the Condor Galaxy AI supercomputer, our value increases as the size of the problem gets larger and larger and larger.”
About the Author
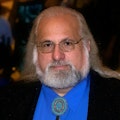