Executive Roundtable: Evolution of Data Center SLAs in the Age of AI
For the second installment of our Executive Roundtable for the Third Quarter of 2024, we asked our four seasoned data center industry leaders how they see service level agreements (SLAs) evolving for data center equipment and expansion projects in the age of rapidly escalating AI, HPC and cloud computing demand.
When implementing data center colocation services, traditionally one of the most important elements to analyze is the SLA a provider offers. This all-encompassing document defines and outlines the level of support and services customers can expect, ensuring a concrete agreement between the provider and the client.
However, SLAs in the age of AI and cloud ubiquity stand to become more complex, and must be examined closely to guarantee a firm understanding of what is being offered and what the customer will see as a part of services.
Our Executive Roundtable for the Third Quarter of 2024 brings together data center industry leaders including:
Danielle Rossi, Global Director – Mission Critical Cooling, Trane
Sean Farney, VP of Data Center Strategy for the Americas, JLL
Harry Handlin, U.S. Data Center Segment Leader, ABB
Josh Claman, CEO, Accelsius
Let's now look into the second question of the series for our Executive Roundtable for the Third Quarter of 2024.
Data Center Frontier: How do you see service level agreements (SLAs) evolving for data center equipment and expansion projects in the age of rapidly escalating AI, HPC and cloud computing demand?
Danielle Rossi, Trane: With the increase of AI, we are seeing drastic rises in density.
Supporting high densities during outages and maintenance requires extra redundancy and planning.
Going forward, we will see more stringent plans for servicing, including connected and virtual services, as well as long-term vendor-provided scheduled maintenance.
Customers have already begun specifying longer-range service plans and more detailed spare parts kits.
Along with these requirements, requests for vendor service capabilities are increasing.
Global technician coverage and area-specific availability have always been a consideration but are increasing in priority.
Sean Farney, JLL: I've managed to SLAs across all levels of infrastructure for products ranging from low-latency trading to natural gas to Happy Meal toys (seriously!), and it's quite operationally complex.
On top of the complexity, appetite for technical downtime is plunging as connectedness becomes more pervasive and we trust digital infrastructure to deliver more mission-critical services related to health, safety, security, finance, autonomous vehicles and more.
I think service level expectations and requirements will continue to creep upward.
To satisfy this need and reduce the brand risk and costs of violation, I see a surge in reliability engineering.
This means taking a systemic approach to designing, building and operating technical infrastructure to higher uptime levels instead of cobbling together services for individual mechanical, electrical and plumbing (MEP) and information technology (IT) components.
JLL's Reliability Engineering practice is inundated with requests to solve these big, impactful problems.
The long-term, holistic approach leads to better performance, lower cost and smoother operations.
Harry Handlin, ABB: Innovation will be the key driver in the evolution of service level agreements for infrastructure equipment.
AI presents many challenges for service organizations.
First, the rapid growth of the AI market coupled with the increased scale of data centers has created a shortage of qualified service engineers and technicians.
In addition, AI data center locations are not constrained by latency requirements. This has results in many data centers being in built in areas that are unlikely to be supported by a four-hour response time.
For some remote sites, the location is more than four hours of travel from the closet field service location.
Josh Claman, Accelsius: With AI and large learning models, we frequently hear that it doesn’t really matter if there’s downtime.
No one talks about five-nines or other traditional IT metrics of availability when it comes to LLMs.
The problem, however, is that the assets you’ve deployed can’t sit idle–because, once idle, your ROI for those assets is now muted.
Sure, for inference engines, high-frequency trading and HPCs, we should aim for that 99.999% availability.
But for large learning models, the math needs to change.
You need to ensure those assets are fully utilized, which is why a lot of our clients move to liquid cooling.
They want to avoid the throttling events that occur with traditional air cooling.
Futuristic AI data center rendering. Does this image envision some future prospect where AI travels holographically for SLA via the visible light spectrum? Probably not. And yet, gazing into the GPUs' illustrated self-conception, such questions arise.
Next: Achieving Balance in AI Data Center Designs
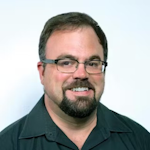
Matt Vincent
A B2B technology journalist and editor with more than two decades of experience, Matt Vincent is Editor in Chief of Data Center Frontier.