NVIDIA Beefs Up Data Center GPUs, Teams with ARM on IoT Devices
As artificial intelligence workloads grow, NVIDIA continues to bring more computing horsepower to crunch it. NVIDIA today introduced beefier GPUs, along with a new interconnect fabric to accelerate workloads, and an initiative to extend machine learning capabilities to smartphones and Internet of Things (IoT) devices.
At a keynote speech at NVIDIA”s GPU Technology Conference in Santa Clara, CEO Jensen Huang unveiled a series of enhancements to the company’s GPU platform for the data center, including a memory boost for its flagship Tesla V100 datacenter GPU, the NVIDIA NVSwitch interconnect fabric, and updates to the company’s software stack.
Huang also showcased what he called “the world’s largest GPU” – an upgraded version of NVIDIA’s DGX “supercomputer in a box.” The NVIDIA DGX-2 server can deliver two petaflops of computational power, using the NVSwitch fabric to connect 16 of the upgraded V100 GPUs, now each with 32 GBs of memory. NVIDIA said the DGX-2 has the deep learning processing power of 300 servers occupying 15 racks of datacenter space, all in a single enclosure with a power footprint of about 10kW.
“We are dramatically enhancing our platform’s performance at a pace far exceeding Moore’s law, enabling breakthroughs that will help revolutionize healthcare, transportation, science exploration and countless other areas,” said Jensen Huang, NVIDIA founder and CEO.
NVIDIA also announced plans to partner with ARM to bring deep learning inferencing to the billions of smartphones, tablets and Internet of Things devices that will enter the global marketplace.
Despite all the announcements of enhancements for the data center and IoT, Wall Street was focused on NVIDIA’s technology for self-driving cars in the wake of a fatility involving an Uber autonomous vehicle. Shares of NVIDIA sold off sharply , closing 7 percent lower after Reuters reported that the company said it was “temporarily suspending the testing of its self-driving cars on public roads to learn from the Uber incident.”
During his keynote, Huang highlighted NVIDIA Drive Sim, which creates virtual reality simulations to test self-driving vehicles, a segment of the market that could benefit if regulators seek to limit on-road test driving of autonomous vehicles.
Tesla V100 Gets Double the Memory
NVIDIA’s graphics processing (GPU) technology has been one of the biggest beneficiaries of the rise of specialized computing, gaining traction with workloads in supercomputing, artificial intelligence (AI) and connected cars. NVIDIA has been investing heavily in innovation in AI, which it sees as a pervasive technology trend that will bring its GPU technology into every area of the economy and society.
The GTC18 conference provided a platform for NVIDIA to showvcase the latest improvements in its hardware and software. At the heart of this effort is the Volta architecture, which is now offered by every major computer vendor and cloud service to deliver artificial intelligence and high performance computing.
The Tesla V100 GPU has received a memory boost from 16GB to 32GTB, which will help data scientists train deeper and larger deep learning model, and improve the performance of memory-constrained HPC applications.
“With the new Tesla V100 32GB GPUs, we will be able to train larger, more complex AI models faster,” said Xuedong Huang, technical fellow and head of speech and language at Microsoft. “This will help extend the accuracy of our models on speech recognition and machine translation reaching human capabilities and enhancing offerings such as Cortana, Bing and Microsoft Translator.”
HPC vendors Cray, Hewlett Packard Enterprise, IBM, Lenovo, Supermicro and Tyan all announced they will begin rolling out Tesla V100 32GB systems within the second quarter, while Oracle Cloud Infrastructure plans to offer them in the cloud in the second half of the year.
A Faster Fabric
NVIDIA says the faster interconnect in its NVSwitch will allow developers to build systems with more GPUs connected to each other, creating systems that can run much larger datasets. It also opens the door to larger, more complex workloads, including modeling parallel training of neural networks.
The NVIDIA NVSwitch was unveiled Tuesday at the NVIDIA GTC conference. (Image: NVIDIA)
Interconnects are network components that allow compute nodes to communicate with each other. Ethernet and Infiniband have been the leading interconnect technologies in high-performance computing. In 2014 NVIDIA introduced NVLink, an interconnect optimized to connect GPUs to CPUs, or connect nodes in an all-GPU system.
The new NVSwitch succeeds NVLink as the NVIDIA’s pemier interconnect offering. The company says NVSwitch can allow 8 GPU pairs to communicate at 300 GB/second, for total throughput of 2.4 TB /second.
Working With ARM on Inference for IoT
The partnership with ARM, a leading provider of low-power chips for smartphones and mobile devices, offers NVIDIA the opportunity to extend its influence as some machine learning tasks begin to take place on edge-based devices, rather than in massive data centers. We’ve previously noted the development of new chips and devices moving more workloads and tasks to the very edge of the network. This trend is expected to play a leading role in the Internet of Things, but is also emerging as a key strategy in artificial intelligence, providing the ability to run neural networks on smartphones.
Under the partnership announced at GTC18, NVIDIA and Arm will integrate the open-source NVIDIA Deep Learning Accelerator (NVDLA) architecture into Arm’s Project Trillium platform for machine learning. The collaboration will make it simpler for IoT chip companies to integrate AI into their designs.
“Accelerating AI at the edge is critical in enabling Arm’s vision of connecting a trillion IoT devices,” said Rene Haas, executive vice president, and president of the IP Group, at Arm. “Today we are one step closer to that vision by incorporating NVDLA into the Arm Project Trillium platform, as our entire ecosystem will immediately benefit from the expertise and capabilities our two companies bring in AI and IoT.”
It’s helpful to note that there are two primary types of computing workloads for machine learning. In training, the network learns a new capability from existing data. In inference, the network applies its capabilities to new data, using its training to identify patterns and perform tasks, usually much more quickly than humans could. Training is a compute-intensive process best suited for data centers, while inference is beginning to shift to devices.
“Inferencing will become a core capability of every IoT device in the future,” said Deepu Talla, vice president and general manager of Autonomous Machines at NVIDIA. “Our partnership with Arm will help drive this wave of adoption by making it easy for hundreds of chip companies to incorporate deep learning technology.”
NVIDIA was founded in 1993, and its graphics processing units (GPUs) quickly became an essential tool for gamers yearning for more horsepower. The company’s GPUs worked with CPUs, but took a slightly different approach to processing data. A CPU consists of a few cores optimized for sequential serial processing, while a GPU has a parallel architecture consisting of hundreds or even thousands of smaller cores designed for handling multiple tasks simultaneously.
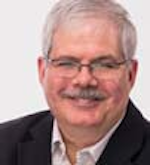