Meta's preview of its newest data center technology highlights how artificial intelligence is changing digital infrastructure. At its AI Infra @ Scale event, Meta showed off custom chips that will boost its ability to process the generative AI workloads that have become all the hotness in recent months.
Meta's custom silicon program reflects the ongoing shift from general purpose CPUs to domain-specific silicon optimized for specific workloads. It's a trend that has been underway for some time, as Meta joins hyperscale cohorts Google, Microsoft and Amazon Web Services in using in-house hardware to build more powerful and efficient cloud infrastructure.
The compute-heavy nature of AI will intensify the need for domain-specific chips for anyone who seeks to compete at scale. On Thursday, Meta introduced two new in-house chips targeting workloads central to the AI boom.
- The Meta Training and Inference Accelerator (MTIA) is an inference accelerator that will enable faster processing of compute-intensive features in the AI services that Meta builds for its users.
- The Meta Scalable Video Processor (MSVP) will accelerate live-streaming and video on demand (VOD) content that users create, including new types of content produced through generative AI.
Meta says that building its own chips will offer granular improvements in performance, power efficiency and cost when they are deployed in 2025.
"By having it in-house we are able to optimize every single nanometer of the chip," said Olivia Wu, Technical Lead for Infra Silicon at Meta. "So we don't have any part of the architecture that is wasted and that helps to bring down the power for the chip. That effectively reduces the cost for the ASIC."
"The benefit of building our own in-house ASICs is that we have access to the real workloads that are used by our ads team and other groups here so we can perform performance analysis on our design," said Linda Cheng, Asic Engineer, Infra Silicon at Meta. "Through this process, we can analyze and fine tune and tweak all the parameters that go into a high- performance solution."
The Case for Domain-Specific Silicon
In artificial intelligence (AI), computers are assembled into neural networks that emulate the learning process of the human brain to solve new challenges. It’s a process that requires lots of computing horsepower, which is why the leading players in the field have moved beyond traditional CPU-driven servers, primarily to GPUs (graphics processing units). A CPU consists of a few cores optimized for sequential serial processing, while a GPU has a parallel architecture consisting of hundreds or even thousands of smaller cores designed for handling multiple tasks simultaneously, an approach which has proven effective on AI workloads.
Meta has been building custom hardware using GPUs since 2016. But in 2020 it began working on its own custom chips.
"We found that GPUs were not always optimal for running Meta’s specific recommendation workloads at the levels of efficiency required at our scale," the Meta Engineering team wrote in a blog post. "Our solution to this challenge was to design a family of recommendation-specific Meta Training and Inference Accelerator (MTIA) ASICs. We co-designed the first-generation ASIC with next-generation recommendation model requirements in mind and integrated it into PyTorch (Meta's open source machine learning framework) to create a wholly optimized ranking system."
The MTIA accelerator is fabricated at TSMC (Taiwan Semiconductor) using a 7nm process and runs at 800 MHz, with a thermal design power (TDP) of 25 W. It uses the RISC-V instruction set architecture (ISA), an open source alternative to the x86 and ARM architectures,
To examine how Meta will use the MTIA, it's helpful to understand the two primary types of AI computing tasks.
- In training, the network learns a new capability from existing data. Training is compute-intensive, requiring hardware that can process huge volumes of data.
- In inference, the network applies its capabilities to new data, using its training to identify patterns and perform tasks much more quickly than humans could.
After being launched to accelerate the recommendation engine for Facebook's newsfeed, the newest version of the MTIA is tailored to perform inference for generative AI workloads.
"Our recommender models were traditionally memory or network bound," said Alexis Bjorlin, VP of Engineering Infrastructure at Meta. "Generative AI is incredibly compute intensive, so the compute density needs to increase."
Running a compute-heavy architecture is super expensive, which also means that small refinements can bring savings as well as efficiency.
"These are also incredibly capital-intensive system," said Bjorlin. "That's one of the main reasons we have the opportunity to innovate end-to-end and that's one of the reasons we kicked off our internal custom silicon development so that we could optimize our specific clusters, our specific infrastructure to meet the efficiency, performance, power and capital efficiency required for the evolution of AI workloads."
Generative AI Will Bring More Video
The MSVP chip will specialize in encoding video content in both production and delivery. That's already a huge challenge for Facebook, which serves more than 4 billion video views per day
"Processing video for video on demand (VOD) and live streaming is already compute intensive," wrote Meta engineers Harikrishna Reddy, Yunqing Chen in a blog post introducing the MSVP chip. "It involves encoding large video files into more manageable formats and transcoding them to deliver to audiences. Today, demand for video content is greater than ever. And emerging use cases, such as generative AI content, mean the demands on video infrastructure are only going to intensify.
"GPUs and even general-purpose CPUs are capable of handling video processing," they added. "But at the scale that Meta operates (serving billions of people all over the world), and with an eye on future AI-related use cases, we believe that dedicated hardware is the best solution in terms of compute power and efficiency."
The MSVP is also an in-house-developed ASIC solution, and can be configured to efficiently support both the high-quality transcoding needed for VOD as well as the low latency and faster processing times that live streaming requires.
Here's a video that provides more details on MSVP.
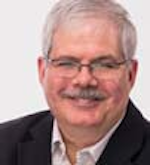