What Does it Really Take to Be AI Ready in a GPT Era?
When AFCOM released its first-ever State of the Data Center report in 2016, the average rack density reported by survey respondents was 6.1 kW. It took eight years for average rack densities to double. Then came the adoption of generative AI, and density rose 3x in a year. (Nvidia’s 2024-era GPU architecture, Hopper, was designed for a density of 41 kW per rack; the very next generation, Blackwell, is architected for 120 kW.) Two years from now, density will have risen another 5x (in March, Nvidia CEO Jensen Huang announced a roadmap for 600 kW racks by the end of 2027).
Generative AI isn’t our grandparents’ neural network. In 2018, the introduction of generative pre-trained transformers (GPTs), a type of large language model (LLM), represented a breakthrough in AI innovation. For the first time ever, AI was able to not only analyze and process information but also create original content. Today, applications like ChatGPT make generative AI accessible to anyone with an internet connection. Adoption has been widespread, and fast.
Still, the rate and scale of generative AI usage isn’t the only thing driving change in IT deployments. It’s also the computational intensity of the training models and the size of the GPU clusters. A GPT model’s capability depends in part on the number of parameters it is trained on, and they’ve grown from a hundred million parameters in 2018 to well over a trillion today. Before transformers, AI models’ computational requirements grew 8x every two years; now they’re growing 256x.
To carry out increasingly intensive training, GPTs’ clusters of connected GPUs are getting larger. For example, Meta’s model Llama 3.1 405B was trained on 16,000 NVIDIA H100 GPUs, and its newest model, Llama 4, is reportedly being trained on a cluster ten times larger (“bigger than 100,000 H100 GPUs,” Meta CEO Mark Zuckerberg has said). The scale and pace of growth defies what was previously thought possible.
But what about DeepSeek? Yes, the Chinese AI company claims to have created a sophisticated machine learning model with a fraction of the resources major U.S. AI companies use. If the claims are true (it seems DeepSeek may have benefited from a distillation of ChatGPT), it could mean that training more sophisticated models will not take as much power as we all thought. But the odds that this will meaningfully reduce exponential demand growth are slim; if history is any indication, the companies advancing AI models will use the efficiency gains to do more. (This is Jevons paradox. As Microsoft CEO Satya Nadella put it, “as AI gets more efficient and accessible, we will see its use skyrocket, turning it into a commodity we just can't get enough of.”)
Ultimately, the impact of generative AI on data centers is profound, driving higher densities and bigger campuses (more land and more power), all with a much higher rate of velocity than ever before. It begs two questions:
- Given that data centers take years to develop, how can a data center provider support technologies that change every 12 months or faster?
- How does a data center built today not become obsolete in just a few years?
For Smart Developers, The More Things Change, The More They Stay the Same
When a data center developer prioritizes proactivity with a focus on configurable designs and replicable (but adaptable) processes, facilities can be built to accommodate change without losing time, resources or value when pivots are needed.
Every developer has their own approach to accomplishing that. For us, it’s about staying focused on the strong fundamentals that have stood the test of time: proactive developments, rationalized supply chains, collaborative innovation and design flexibility.
1. Proactive – not speculative – power and land development is the best primer.
To reduce uncertainty and ensure crucial capacity is available when and where it’s needed, the largest data center users are trying to shorten the time between when demand is confirmed and when capacity comes online. By proactively securing power and developing de-risked land for potential build-to-suit deployments, we enable customers to defer decisions about how much capacity they’ll need, where and in what configuration until they know more about their actual demand.
This is very different than the kind of purely speculative development causing strain in utility queues and complicating the market for real providers and end users (we did a deep dive on this “age of fake data centers” in our last article). In contrast, proactive development relies on deep relationships with utilities, municipal leaders and customers — along with an extremely technical and rigorous approach to site selection.
Here, a detailed and standardized assessment framework backed by proprietary GIS tools is our key to uncovering gold in a crowded national landscape. The wide variety of data we assess — power transmission lines as just one example out of hundreds — provides us with a significantly deeper view than most developers take. Meanwhile, site selection software covering 14 domains, each with dozens of line items, layers comprehensive data over the markets. This kind of software enables us to expedite our desktop diligence processes, giving us more time to invest in our relationships with local utilities and municipal leaders and ensure a clearer pathway to the kinds of permits and power AI-ready campuses require.
If everyone can stick to proactive development in alignment with real demand and stay away from purely speculative moves, the market can remain more agile and potentially help avoid some bottlenecks we see forming. But the best developers using the right tools will be able to find de-risked, AI-ready sites regardless of how their peers proceed.
2. A kit of parts, well-developed supply chain and OFCI practices keep processes flowing.
Data center development processes aren’t the most agile, but in today’s climate of innovation, they must have flexibility built in. A standardized, replicable kit of parts that still allows for requirement-specific configuration, combined with a well-developed supply chain and OFCI (owner-furnished, contractor-installed) practices is the best way forward.
When the COVID-19 pandemic upended global supply chains, we standardized our data center deployments across our portfolio with a design that meets the building and performance specifications of the world’s largest data center users. Having a standardized MEP package enables us to aggregate our equipment supply so we’re not beholden to manufacturers’ supply chains. We’re also able to deploy the MEP equipment where and when it’s needed to meet demand across our portfolio.
A standardized kit of parts de-risks procurement of the longest lead equipment to stay ahead of demand and gives a speed boost. As a result, we’re able to deliver on time for customers even when supply chains are disrupted. It also means we can give customers the ability to defer or modify decisions (e.g., about what kind of cooling system they need) without extending time to capacity. That kind of wiggle room has proven invaluable in this era of massive and rapid technological advancement.
3. Collaborative innovation makes developments and designs nimble.
More than ever, being an innovative data center developer means keeping our fingers on the pulse of technology change and being in the rooms where innovations are taking place. Like at OCP, where we’re one of the few data center providers in the room with the manufacturers whose hardware innovations are driving the need for liquid cooling. Maintaining agility also requires trust-based relationships that give end users the confidence to share their future requirements with their data center developer. At Stream, for example, 25+ years of tenant relationships and a reputation for trustworthiness enabled us to proactively collaborate on our next-generation cooling system to better support tenants’ AI deployments.
Most assumed AI would change the game, but the reality of that change was still fuzzy for many. Thanks to our relationships and insights, we saw the AI sea change coming and the resulting need for direct liquid cooling. We also saw that there was no off-the-shelf solution that would accommodate traditional and high-density AI deployments without extensive rework and added expense. So, working closely with our customers, we developed a configurable and innovative proprietary direct liquid cooling (DLC) design that supports both air cooling and liquid to the rack with lower costs and less supply chain risk than off-the-shelf DLC solutions. It also helps facilities transition between modalities without stranding air cooling resources.
This pre-engineered integrated solution, the STU (Server Thermal Unit), supports Nvidia’s generative AI architecture, Blackwell, right out of the box. And because we design our innovations to be backwards-compatible, they’ll support customers’ technological advancements for years to come. Doing both is no easy feat; developers must work harder to develop solutions that offer both standardization and flexibility to accommodate requirements that may differ from one tenant to the next, or even from one day to the next. This kind of adaptability is particularly essential as AI capabilities — and the resulting data center requirements — rapidly evolve.
Trust and collaboration are what give you access to the information you need as soon as it’s available, which is vital for staying ahead of change instead of falling victim to it. When driving innovation in close alignment with customer requirements and business decisions, it’s far easier to balance evolution with precision — and precision with flexibility.
Generative AI is a technological sea change that could be as consequential as the printing press, the steam engine or electricity. But while it took decades or even centuries to achieve widespread adoption of those technologies, generative AI has become practically ubiquitous in just two years. At this pace of change, no one can predict the exact future. However, for data center developers, being AI ready in a GPT era means being proactive, collaborative and flexible to support what is ahead — and cultivating relationships that help you see what’s coming before it blindsides you.
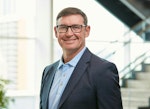
Stuart Lawrence
Stuart Lawrence is VP of Product Innovation & Sustainability at Stream Data Centers – which builds and operates data centers for the largest and most sophisticated enterprises and hyperscalers – since 1999, celebrating our Silver Anniversary with 90% of our capacity leased to the Fortune 100.